AI method identifies diseases faster than humans.
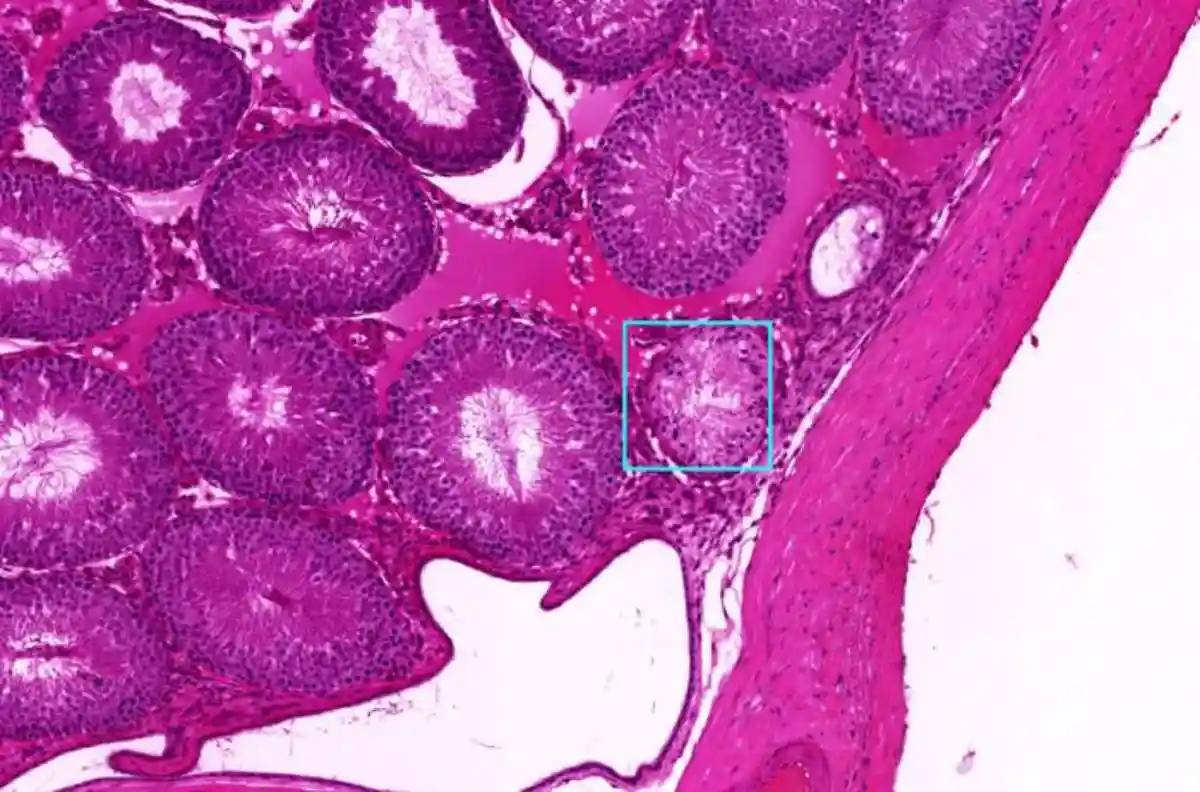
A deep learning-based artificial intelligence model developed at Washington State University can quickly and often more accurately detect signs of disease in images of animal and human tissue compared to human assessments.
As reported in Scientific Reports, this innovation could significantly accelerate disease-related research. It also offers promising advancements for medical diagnostics, enabling, for instance, the detection of cancer in biopsy images within minutes—a task that would usually take human pathologists several hours.
“This AI-based deep learning program was very, very accurate at looking at these tissues,” said Michael Skinner, a WSU biologist and co-corresponding author on the paper. “It could revolutionize this type of medicine for both animals and humans, essentially better facilitating these kinds of analysis.”
To develop the AI model, computer scientist Colin Greeley, a former graduate student at WSU, and his advisor, Professor Lawrence Holder, trained it using images from past epigenetic studies conducted in Professor Skinner’s lab. These studies focused on molecular-level indicators of disease in rat and mouse kidney, testes, ovarian, and prostate tissues.
The team then tested the model on images from other research, including studies on breast cancer and lymph node metastasis. They discovered that the AI model not only identified pathologies accurately and quickly but also outperformed previous models in speed. Remarkably, it even detected instances that a team of trained human experts had overlooked.
“I think we now have a way to identify disease and tissue that is faster and more accurate than humans,” said Holder, a co-corresponding author on the study.
Traditionally, this type of tissue analysis required labor-intensive work from specialized teams who examined and annotated slides under a microscope, often double-checking each other’s work to minimize human error. In Professor Skinner’s epigenetic research—focused on studying changes in molecular processes that affect gene behavior without altering the DNA itself—this process could take a year or more for large studies. With the new AI deep learning model, the same analysis can now be completed within weeks, Skinner noted.
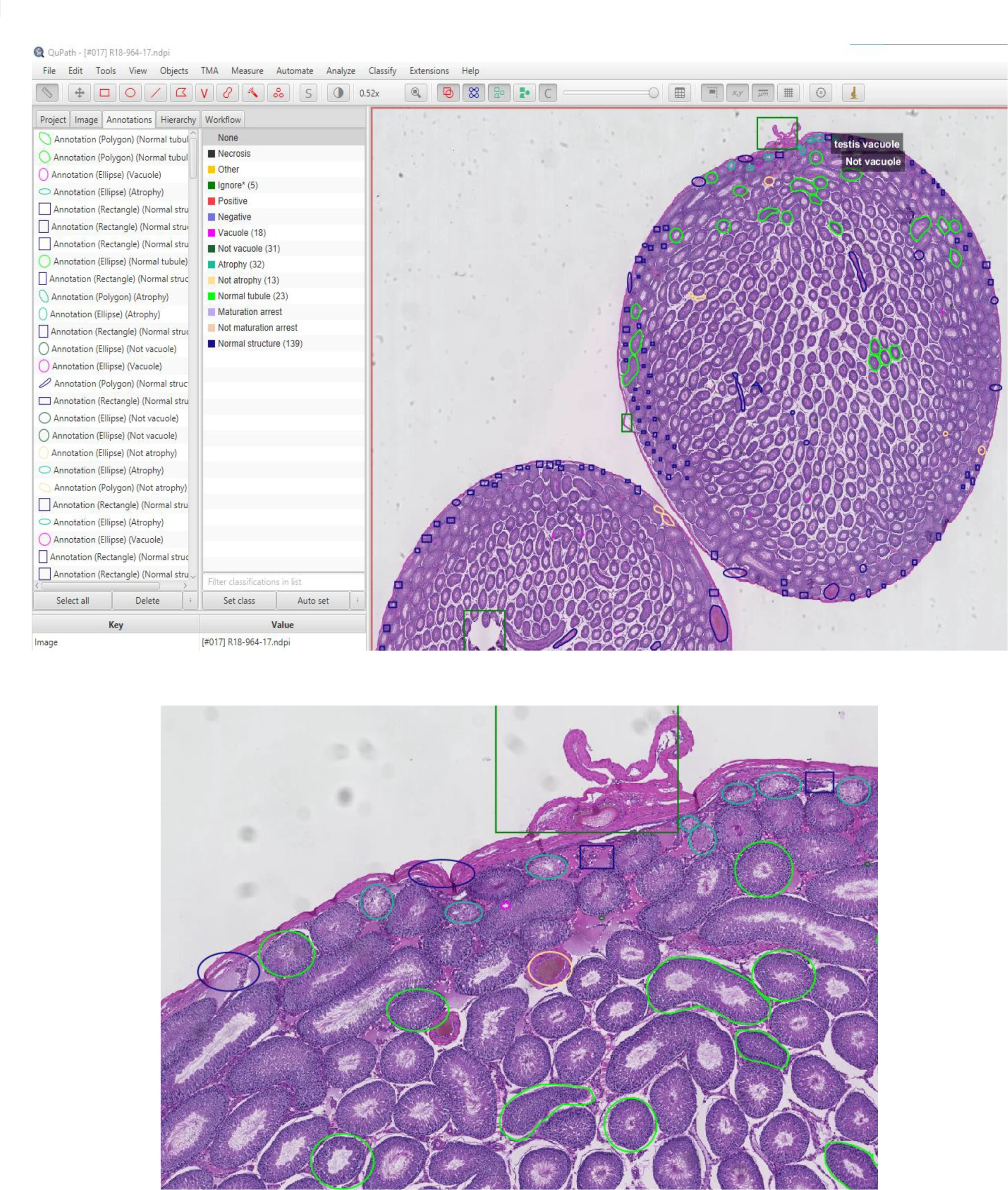
Deep learning, an advanced AI method aiming to mimic the human brain, goes beyond traditional machine learning, explained Holder. Unlike basic machine learning, a deep learning model is built with a network of neurons and synapses that allow it to “learn” from errors through a process called backpropagation. This process adjusts the network after mistakes, helping the model avoid repeating them in the future.
The WSU team designed their deep learning model to work with extremely high-resolution gigapixel images, which contain billions of pixels. Because such large files can overwhelm even high-performance computers, the researchers developed the model to analyze smaller, individual tiles of the image while still referencing the larger context at a lower resolution—similar to zooming in and out with a microscope.
The new model is already gaining attention from other researchers. Holder’s team is currently collaborating with WSU veterinary scientists to diagnose disease in tissue samples from deer and elk. The authors also highlight the model’s potential in advancing human research and diagnosis, especially for cancer and other gene-related diseases. As long as annotated data is available, such as images identifying cancer in tissues, the AI model can be trained to perform this work, said Holder.
“The network that we’ve designed is state-of-the-art,” Holder said. “We did comparisons to several other systems and other data sets for this paper, and it beat them all.”
The study was supported by the John Templeton Foundation, with Eric Nilsson, a research assistant professor at WSU’s School of Biological Sciences, contributing as a co-author on the paper.